极端曝光图像的多尺度分解细节感知融合算法
2024,46(2):162-173
张俊超
中南大学 自动化学院, 湖南 长沙 410083,junchaozhang@csu.edu.cn
黄俊彬
中南大学 自动化学院, 湖南 长沙 410083
杨德贵
中南大学 自动化学院, 湖南 长沙 410083,degui.yang@csu.edu.cn
梁步阁
中南大学 自动化学院, 湖南 长沙 410083
陈溅来
中南大学 自动化学院, 湖南 长沙 410083
赵党军
中南大学 自动化学院, 湖南 长沙 410083
中南大学 自动化学院, 湖南 长沙 410083,junchaozhang@csu.edu.cn
黄俊彬
中南大学 自动化学院, 湖南 长沙 410083
杨德贵
中南大学 自动化学院, 湖南 长沙 410083,degui.yang@csu.edu.cn
梁步阁
中南大学 自动化学院, 湖南 长沙 410083
陈溅来
中南大学 自动化学院, 湖南 长沙 410083
赵党军
中南大学 自动化学院, 湖南 长沙 410083
摘要:
针对极端曝光(欠曝光和过曝光)图像动态范围低的问题,提出一种基于多尺度分解细节感知的图像融合算法。欠曝光图像经过细节增强后,与过曝光图像进行初步的粗融合;采用小波变换对细节增强后图像的亮度分量进行多尺度分解,并设计专门的高频和低频融合策略,实现亮度分量的精融合;将粗融合图像的色调、饱和度分量,与精融合图像的亮度分量重组,获得最终的融合结果。基于大量测试数据,实验结果表明所提方法在视觉效果方面表现优异,平均MEF-SSIM指标为0.985 4,平均SSIM指标为0.650 8,均优于现有主流算法。
针对极端曝光(欠曝光和过曝光)图像动态范围低的问题,提出一种基于多尺度分解细节感知的图像融合算法。欠曝光图像经过细节增强后,与过曝光图像进行初步的粗融合;采用小波变换对细节增强后图像的亮度分量进行多尺度分解,并设计专门的高频和低频融合策略,实现亮度分量的精融合;将粗融合图像的色调、饱和度分量,与精融合图像的亮度分量重组,获得最终的融合结果。基于大量测试数据,实验结果表明所提方法在视觉效果方面表现优异,平均MEF-SSIM指标为0.985 4,平均SSIM指标为0.650 8,均优于现有主流算法。
基金项目:
微系统技术重点实验室开发课题基金资助项目(6142804231001);国家自然科学基金资助项目(62105372);湖南省自然科学基金资助项目(2021JJ40794)
微系统技术重点实验室开发课题基金资助项目(6142804231001);国家自然科学基金资助项目(62105372);湖南省自然科学基金资助项目(2021JJ40794)
Multi-scale decomposition based detail perception fusion algorithm for extreme exposure images
ZHANG Junchao
School of Automation, Central South University, Changsha 410083, China,junchaozhang@csu.edu.cn
HUANG Junbin
School of Automation, Central South University, Changsha 410083, China
YANG Degui
School of Automation, Central South University, Changsha 410083, China,degui.yang@csu.edu.cn
LIANG Buge
School of Automation, Central South University, Changsha 410083, China
CHEN Jianlai
School of Automation, Central South University, Changsha 410083, China
ZHAO Dangjun
School of Automation, Central South University, Changsha 410083, China
School of Automation, Central South University, Changsha 410083, China,junchaozhang@csu.edu.cn
HUANG Junbin
School of Automation, Central South University, Changsha 410083, China
YANG Degui
School of Automation, Central South University, Changsha 410083, China,degui.yang@csu.edu.cn
LIANG Buge
School of Automation, Central South University, Changsha 410083, China
CHEN Jianlai
School of Automation, Central South University, Changsha 410083, China
ZHAO Dangjun
School of Automation, Central South University, Changsha 410083, China
Abstract:
Aiming at the problem of the low dynamic range of extreme exposure images, such as underexposure and overexposure images, a detail perception image fusion algorithm based on multi-scale decomposition was proposed. After detail enhancement, the underexposed image was roughly fused with the overexposed image. Wavelet transform was used to perform multi-scale decomposition on the brightness component of the image after detail enhancement, and a special high-frequency and low-frequency fusion strategy was designed to realize the fine fusion of brightness components. The hue and saturation components of the coarse fusion image were recombined with the brightness components of the fine fusion image to obtain the final fusion result. Based on abundant testing data, the experimental results show that the visual effect of this method is excellent. The index of averaged MEF-SSIM is 0.985 4, and the index of averaged SSIM is 0.650 8, which are superior to the existing mainstream algorithms.
Aiming at the problem of the low dynamic range of extreme exposure images, such as underexposure and overexposure images, a detail perception image fusion algorithm based on multi-scale decomposition was proposed. After detail enhancement, the underexposed image was roughly fused with the overexposed image. Wavelet transform was used to perform multi-scale decomposition on the brightness component of the image after detail enhancement, and a special high-frequency and low-frequency fusion strategy was designed to realize the fine fusion of brightness components. The hue and saturation components of the coarse fusion image were recombined with the brightness components of the fine fusion image to obtain the final fusion result. Based on abundant testing data, the experimental results show that the visual effect of this method is excellent. The index of averaged MEF-SSIM is 0.985 4, and the index of averaged SSIM is 0.650 8, which are superior to the existing mainstream algorithms.
收稿日期:
2022-04-12
2022-04-12
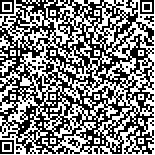