中心约束对比学习的自集成卫星异常检测方法
2024,46(6):33-42
郭国航
中国科学院国家空间科学中心, 北京 100190;
中国科学院大学, 北京 100049,gghnssc@163.com,hutai@nssc.ac.cn
李虎
中国科学院国家空间科学中心, 北京 100190
刘玉荣
中国科学院国家空间科学中心, 北京 100190
胡钛
中国科学院国家空间科学中心, 北京 100190,gghnssc@163.com,hutai@nssc.ac.cn
中国科学院国家空间科学中心, 北京 100190;
中国科学院大学, 北京 100049,gghnssc@163.com,hutai@nssc.ac.cn
李虎
中国科学院国家空间科学中心, 北京 100190
刘玉荣
中国科学院国家空间科学中心, 北京 100190
胡钛
中国科学院国家空间科学中心, 北京 100190,gghnssc@163.com,hutai@nssc.ac.cn
摘要:
针对现有异常检测算法对遥测参数特征提取缺乏区分度以及异常决策信息丢失等问题,提出基于中心约束对比的自集成异常检测方法。融合对比损失和中心损失将正常样本映射到紧凑的特征分布,并采用多视角、多层次特征集成的方式实现样本的异常检测,提升了模型对卫星复杂工况的适应性。采用科学卫星真实遥测参数数据和基准数据集进行验证,结果表明,所提方法在真实遥测参数上比最优基准方法的F值提升21.8%,且具有更好的噪声抗干扰性。实验结果验证了方法的可行性,能够为卫星地面运管提供有效的判读支持。
针对现有异常检测算法对遥测参数特征提取缺乏区分度以及异常决策信息丢失等问题,提出基于中心约束对比的自集成异常检测方法。融合对比损失和中心损失将正常样本映射到紧凑的特征分布,并采用多视角、多层次特征集成的方式实现样本的异常检测,提升了模型对卫星复杂工况的适应性。采用科学卫星真实遥测参数数据和基准数据集进行验证,结果表明,所提方法在真实遥测参数上比最优基准方法的F值提升21.8%,且具有更好的噪声抗干扰性。实验结果验证了方法的可行性,能够为卫星地面运管提供有效的判读支持。
基金项目:
中国科学院战略性先导科技专项资助项目(XDA15040100)
中国科学院战略性先导科技专项资助项目(XDA15040100)
Self-ensemble satellite anomaly detection method for center-constrained contrastive learning
GUO Guohang
National Space Science Center, Chinese Academy of Sciences, Beijing 100190, China;
University of Chinese Academy of Sciences, Beijing 100049, China,gghnssc@163.com,hutai@nssc.ac.cn
LI Hu
National Space Science Center, Chinese Academy of Sciences, Beijing 100190, China
LIU Yurong
National Space Science Center, Chinese Academy of Sciences, Beijing 100190, China
HU Tai
National Space Science Center, Chinese Academy of Sciences, Beijing 100190, China,gghnssc@163.com,hutai@nssc.ac.cn
National Space Science Center, Chinese Academy of Sciences, Beijing 100190, China;
University of Chinese Academy of Sciences, Beijing 100049, China,gghnssc@163.com,hutai@nssc.ac.cn
LI Hu
National Space Science Center, Chinese Academy of Sciences, Beijing 100190, China
LIU Yurong
National Space Science Center, Chinese Academy of Sciences, Beijing 100190, China
HU Tai
National Space Science Center, Chinese Academy of Sciences, Beijing 100190, China,gghnssc@163.com,hutai@nssc.ac.cn
Abstract:
To deal with the problem of the existing telemetry anomaly detection algorithms, such as the poor discrimination capability of the feature, and loss of anomaly decision-making information, a self-ensemble anomaly detection method based on center-constrained contrastive learning was proposed. The method mapped the normal samples to a compact feature distribution by combining contrastive loss and center loss, and a multi-view and multi-level ensembled feature decision method was used to obtain the anomaly detection of the sample. The method improves the adaptability of the model to the complex working conditions of the satellite. The real telemetry parameter data of scientific satellite and benchmark data set are used for verification. The proposed method is robust to noise, and achieves 21.8% improvement of F score than that of the state of the art method. The results of the experiment demonstrate the feasibility of the method, which can provide effective support for satellite operation.
To deal with the problem of the existing telemetry anomaly detection algorithms, such as the poor discrimination capability of the feature, and loss of anomaly decision-making information, a self-ensemble anomaly detection method based on center-constrained contrastive learning was proposed. The method mapped the normal samples to a compact feature distribution by combining contrastive loss and center loss, and a multi-view and multi-level ensembled feature decision method was used to obtain the anomaly detection of the sample. The method improves the adaptability of the model to the complex working conditions of the satellite. The real telemetry parameter data of scientific satellite and benchmark data set are used for verification. The proposed method is robust to noise, and achieves 21.8% improvement of F score than that of the state of the art method. The results of the experiment demonstrate the feasibility of the method, which can provide effective support for satellite operation.
收稿日期:
2022-06-28
2022-06-28
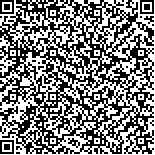