改进YOLOv5s算法在交通标志检测识别中的应用
2024,46(6):123-130
摘要:
针对复杂道路交通场景中交通标志检测识别精度低的问题,提出了一种改进YOLOv5s算法的目标检测识别方法。采用迭代自组织数据分析算法对TT100K数据集进行聚类分析选择更适合的先验框,新先验框能够更加全面地覆盖交通标志的尺寸,提高模型的检测精度;对特征图进行上采样操作,获得更大尺度的特征图后与主干网络特征图融合,得到特征信息更加丰富的新特征图用来小目标的检测识别,提高了小目标检测识别的精度;用真实框和先验框宽比和高比的差值替代真实框和先验框宽高比的差值对定位损失函数进行改进,解决了宽高比相同但实际尺寸不同时惩罚消失问题。实验结果表明改进算法与YOLOv5s算法相比,提高了9.55%的平均精度均值,对小目标的检测识别具有更好的效果。
针对复杂道路交通场景中交通标志检测识别精度低的问题,提出了一种改进YOLOv5s算法的目标检测识别方法。采用迭代自组织数据分析算法对TT100K数据集进行聚类分析选择更适合的先验框,新先验框能够更加全面地覆盖交通标志的尺寸,提高模型的检测精度;对特征图进行上采样操作,获得更大尺度的特征图后与主干网络特征图融合,得到特征信息更加丰富的新特征图用来小目标的检测识别,提高了小目标检测识别的精度;用真实框和先验框宽比和高比的差值替代真实框和先验框宽高比的差值对定位损失函数进行改进,解决了宽高比相同但实际尺寸不同时惩罚消失问题。实验结果表明改进算法与YOLOv5s算法相比,提高了9.55%的平均精度均值,对小目标的检测识别具有更好的效果。
基金项目:
国家自然科学基金青年基金资助项目(61501470)
国家自然科学基金青年基金资助项目(61501470)
Application of improved YOLOv5s algorithm in traffic sign detection and recognition
GUO Junbin
College of Missile Engineering, Rocket Force University of Engineering, Xi′an 710025, China
YU Lin
College of Missile Engineering, Rocket Force University of Engineering, Xi′an 710025, China
YU Chuanqiang
College of Missile Engineering, Rocket Force University of Engineering, Xi′an 710025, China
College of Missile Engineering, Rocket Force University of Engineering, Xi′an 710025, China
YU Lin
College of Missile Engineering, Rocket Force University of Engineering, Xi′an 710025, China
YU Chuanqiang
College of Missile Engineering, Rocket Force University of Engineering, Xi′an 710025, China
Abstract:
Aiming at the problem of low detection and recognition accuracy of traffic signs in complex traffic scenes, a target detection and recognition method based on improved YOLOv5s algorithm was proposed. Iterative self-organizing data analysis techniques algorithm was used for clustering analysis of TT100K data set to select the prior frame which was more suitable for the size of traffic signs. The new prior frame could cover the size of traffic signs more comprehensively and improve the detection accuracy of the model. The feature map was upsampled to obtain a larger scale feature map, and then contacted with the feature map of the backbone network to obtain a new feature map with more abundant feature information. The new feature map was used for small target detection and recognition, which improved the accuracy of small target detection and recognition. And the difference of the width ratio and height ratio between the real frame and the prior frame was used to replace the difference of the aspect ratio between the real frame and the prior frame to improve the positioning loss function, which solved the problem of penalty disappearing when the width ratio was the same but the actual size was different. Experimental results show that compared with the original YOLOv5s algorithm, the improved algorithm can improve the mean average precision by 9.55%, and has better performance in detecting and recognizing small targets.
Aiming at the problem of low detection and recognition accuracy of traffic signs in complex traffic scenes, a target detection and recognition method based on improved YOLOv5s algorithm was proposed. Iterative self-organizing data analysis techniques algorithm was used for clustering analysis of TT100K data set to select the prior frame which was more suitable for the size of traffic signs. The new prior frame could cover the size of traffic signs more comprehensively and improve the detection accuracy of the model. The feature map was upsampled to obtain a larger scale feature map, and then contacted with the feature map of the backbone network to obtain a new feature map with more abundant feature information. The new feature map was used for small target detection and recognition, which improved the accuracy of small target detection and recognition. And the difference of the width ratio and height ratio between the real frame and the prior frame was used to replace the difference of the aspect ratio between the real frame and the prior frame to improve the positioning loss function, which solved the problem of penalty disappearing when the width ratio was the same but the actual size was different. Experimental results show that compared with the original YOLOv5s algorithm, the improved algorithm can improve the mean average precision by 9.55%, and has better performance in detecting and recognizing small targets.
收稿日期:
2022-07-05
2022-07-05
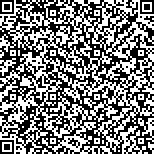