面向辐射源识别的多尺度特征提取与特征选择网络
2024,46(6):141-148
摘要:
目前应用于辐射源识别的卷积神经网络对时序同相正交(in-phase and quadrature-phase,IQ)信号的处理有两种方式:一种方式是将其变换为图像,另一种方式是提取IQ时序数据的浅层特征。前一种方式会导致算法计算量大,而后一种方式会导致识别准确率低。针对上述问题,提出一种多尺度特征提取与特征选择网络。该网络以IQ信号为输入,经多尺度特征提取网络提取IQ信号的浅层特征和多尺度特征,采用特征选择网络降低多尺度特征的数据维度,通过自适应线性整流单元实现特征增强,使用单个全连接层对辐射源进行分类。在FIT/CorteXlab射频指纹识别数据集上,与ORACLE、CNN-DLRF和IQCNet对比实验表明,所提网络在一定程度上提高了识别准确率,降低了计算量。
目前应用于辐射源识别的卷积神经网络对时序同相正交(in-phase and quadrature-phase,IQ)信号的处理有两种方式:一种方式是将其变换为图像,另一种方式是提取IQ时序数据的浅层特征。前一种方式会导致算法计算量大,而后一种方式会导致识别准确率低。针对上述问题,提出一种多尺度特征提取与特征选择网络。该网络以IQ信号为输入,经多尺度特征提取网络提取IQ信号的浅层特征和多尺度特征,采用特征选择网络降低多尺度特征的数据维度,通过自适应线性整流单元实现特征增强,使用单个全连接层对辐射源进行分类。在FIT/CorteXlab射频指纹识别数据集上,与ORACLE、CNN-DLRF和IQCNet对比实验表明,所提网络在一定程度上提高了识别准确率,降低了计算量。
基金项目:
Multi-scale feature extraction and feature selection network for radiation source identification
ZHANG Shunsheng
Research Institute of Electronic Science and Technology, University of Electronic Science and Technology of China, Chengdu 611731, China
DING Huancheng
Research Institute of Electronic Science and Technology, University of Electronic Science and Technology of China, Chengdu 611731, China
WANG Wenqin
School of Information and Communication Engineering, University of Electronic Science and Technology of China, Chengdu 611731, China
Research Institute of Electronic Science and Technology, University of Electronic Science and Technology of China, Chengdu 611731, China
DING Huancheng
Research Institute of Electronic Science and Technology, University of Electronic Science and Technology of China, Chengdu 611731, China
WANG Wenqin
School of Information and Communication Engineering, University of Electronic Science and Technology of China, Chengdu 611731, China
Abstract:
Convolutional neural networks currently applied to radiation source identification process the time-series IQ( in-phase and quadrature-phase) signals in two ways:one way transforms them into images, and the other way extracts shallow features of the IQ time-series data. The former way leads to a large computational effort of the algorithm, while the latter way leads to a low accuracy of the recognition rate. To address the above problems, a multi-scale feature extraction and feature selection network was proposed. After inputting the IQ signal, the shallow and multi-scale features of the IQ signal were extracted by the multi-scale feature extraction network. Then the data dimension of multi-scale features was reduced by the feature selection network. Feature enhancement was achieved by the adaptive linear rectification unit, and a single fully connected layer was used to classify the radiation source. Comparison experiments with ORACLE, CNN-DLRF and IQCNet on the FIT/CorteXlab radio frequency fingerprint recognition dataset show that the proposed network improves the recognition accuracy and reduces the computational effort to some extent.
Convolutional neural networks currently applied to radiation source identification process the time-series IQ( in-phase and quadrature-phase) signals in two ways:one way transforms them into images, and the other way extracts shallow features of the IQ time-series data. The former way leads to a large computational effort of the algorithm, while the latter way leads to a low accuracy of the recognition rate. To address the above problems, a multi-scale feature extraction and feature selection network was proposed. After inputting the IQ signal, the shallow and multi-scale features of the IQ signal were extracted by the multi-scale feature extraction network. Then the data dimension of multi-scale features was reduced by the feature selection network. Feature enhancement was achieved by the adaptive linear rectification unit, and a single fully connected layer was used to classify the radiation source. Comparison experiments with ORACLE, CNN-DLRF and IQCNet on the FIT/CorteXlab radio frequency fingerprint recognition dataset show that the proposed network improves the recognition accuracy and reduces the computational effort to some extent.
收稿日期:
2022-11-28
2022-11-28
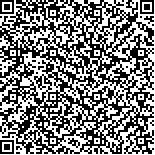