改进协同演化算法求解超多目标云工作流调度问题
2025,47(2):35-48
周佳军
中国地质大学(武汉) 计算机学院, 湖北 武汉 430078
姬小晖
中国地质大学(武汉) 计算机学院, 湖北 武汉 430078
卢超
中国地质大学(武汉) 计算机学院, 湖北 武汉 430078
高亮
华中科技大学 机械科学与工程学院, 湖北 武汉 430074
中国地质大学(武汉) 计算机学院, 湖北 武汉 430078
姬小晖
中国地质大学(武汉) 计算机学院, 湖北 武汉 430078
卢超
中国地质大学(武汉) 计算机学院, 湖北 武汉 430078
高亮
华中科技大学 机械科学与工程学院, 湖北 武汉 430074
摘要:
目前主流优化方法通常将云工作流调度建模为单目标或者不超过三个目标的多目标优化问题,未能充分考虑实际应用场景需求。为克服传统方法局限性,将云工作流调度问题直接建模为涉及时间、费用、可靠性、资源消耗度、负载均衡等众多指标的超多目标优化问题,并针对该问题提出一种改进协同演化算法,利用双阶段策略和多性能指标协同机制有效地平衡解集的收敛性和多样性,提升算法寻优能力。在七类真实工作流实例上的实验表明,所提方法相比现有算法在大多数情况下可找到更好的调度方案。
目前主流优化方法通常将云工作流调度建模为单目标或者不超过三个目标的多目标优化问题,未能充分考虑实际应用场景需求。为克服传统方法局限性,将云工作流调度问题直接建模为涉及时间、费用、可靠性、资源消耗度、负载均衡等众多指标的超多目标优化问题,并针对该问题提出一种改进协同演化算法,利用双阶段策略和多性能指标协同机制有效地平衡解集的收敛性和多样性,提升算法寻优能力。在七类真实工作流实例上的实验表明,所提方法相比现有算法在大多数情况下可找到更好的调度方案。
基金项目:
国家自然科学基金资助项目(51825502,51905198,52175490)
国家自然科学基金资助项目(51825502,51905198,52175490)
Improved co-evolutionary algorithm for solving many-objective cloud workflow scheduling problem
ZHOU Jiajun
School of Computer Science, China University of Geosciences(Wuhan), Wuhan 430078 , China
JI Xiaohui
School of Computer Science, China University of Geosciences(Wuhan), Wuhan 430078 , China
LU Chao
School of Computer Science, China University of Geosciences(Wuhan), Wuhan 430078 , China
GAO Liang
School of Mechanical Science & Engineering, Huazhong University of Science and Technology, Wuhan 430074 , China
School of Computer Science, China University of Geosciences(Wuhan), Wuhan 430078 , China
JI Xiaohui
School of Computer Science, China University of Geosciences(Wuhan), Wuhan 430078 , China
LU Chao
School of Computer Science, China University of Geosciences(Wuhan), Wuhan 430078 , China
GAO Liang
School of Mechanical Science & Engineering, Huazhong University of Science and Technology, Wuhan 430074 , China
Abstract:
Most current studies formulate the cloud workflow scheduling as a single-objective or multi-objective optimization problem with at most three objectives, which is unable to fully meet practical scenarios′ needs. To address the limitations above, many-objective cloud workflow scheduling model was established, where many indicators such as time, cost, reliability, resource consumption, load balancing, were taken into account. Then, an improved co-evolutionary algorithm was introduced to address this problem, where dual-stage search strategy and multi-indicator cooperation mechanism were employed to effectively balance the convergence and diversity of solution set, so as to enhance the search capability of algorithm. Experiments on seven types of real life workflow instances reveal that our proposal outperforms the existing peers and can find better scheduling schemes in most cases.
Most current studies formulate the cloud workflow scheduling as a single-objective or multi-objective optimization problem with at most three objectives, which is unable to fully meet practical scenarios′ needs. To address the limitations above, many-objective cloud workflow scheduling model was established, where many indicators such as time, cost, reliability, resource consumption, load balancing, were taken into account. Then, an improved co-evolutionary algorithm was introduced to address this problem, where dual-stage search strategy and multi-indicator cooperation mechanism were employed to effectively balance the convergence and diversity of solution set, so as to enhance the search capability of algorithm. Experiments on seven types of real life workflow instances reveal that our proposal outperforms the existing peers and can find better scheduling schemes in most cases.
收稿日期:
2024-01-05
2024-01-05
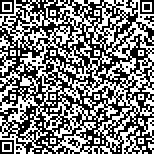