柔性作业车间调度问题的课程强化学习算法
2025,47(2):49-59
卢超
中国地质大学(武汉) 计算机学院, 湖北 武汉 430078
肖洋
中国地质大学(武汉) 计算机学院, 湖北 武汉 430078
张彪
聊城大学 计算机学院, 山东 聊城 252000
高亮
华中科技大学 智能制造装备与技术全国重点实验室, 湖北 武汉 430074
中国地质大学(武汉) 计算机学院, 湖北 武汉 430078
肖洋
中国地质大学(武汉) 计算机学院, 湖北 武汉 430078
张彪
聊城大学 计算机学院, 山东 聊城 252000
高亮
华中科技大学 智能制造装备与技术全国重点实验室, 湖北 武汉 430074
摘要:
针对深度强化学习在柔性作业车间调度问题上泛化能力不足的问题,提出结合课程学习和深度强化学习的方法。通过动态调整训练实例难度,重点增强最难实例的训练,以适应不同数据分布,避免学习过程中的遗忘问题。仿真测试结果表明,算法在未经训练的大规模问题和基准数据集上保持了不错的性能。在2种人造分布的4个未训练大规模问题上取得了更好的性能表现。相较于精确方法和元启发式方法,对于计算量较大的问题实例,能快速地获得质量不错的解。同时算法可以适应不同的数据分布的柔性作业车间调度问题,具有较快收敛速度和较好泛化能力。
针对深度强化学习在柔性作业车间调度问题上泛化能力不足的问题,提出结合课程学习和深度强化学习的方法。通过动态调整训练实例难度,重点增强最难实例的训练,以适应不同数据分布,避免学习过程中的遗忘问题。仿真测试结果表明,算法在未经训练的大规模问题和基准数据集上保持了不错的性能。在2种人造分布的4个未训练大规模问题上取得了更好的性能表现。相较于精确方法和元启发式方法,对于计算量较大的问题实例,能快速地获得质量不错的解。同时算法可以适应不同的数据分布的柔性作业车间调度问题,具有较快收敛速度和较好泛化能力。
基金项目:
国家自然科学基金资助项目(52175490,51805495,52175490);湖北省重点研发计划资助项目(2022BAD121)
国家自然科学基金资助项目(52175490,51805495,52175490);湖北省重点研发计划资助项目(2022BAD121)
Curriculum reinforcement learning algorithm for flexible job shop scheduling problems
LU Chao
School of Computer Science, China University of Geosciences(Wuhan), Wuhan 430078 , China
XIAO Yang
School of Computer Science, China University of Geosciences(Wuhan), Wuhan 430078 , China
ZHANG Biao
School of Computer Science and Technology, Liaocheng University, Liaocheng 252000 , China
GAO Liang
State Key Laboratory of Intelligent Manufacturing Equipment and Technology, Huazhong University of Science and Technology,Wuhan 430074 , China
School of Computer Science, China University of Geosciences(Wuhan), Wuhan 430078 , China
XIAO Yang
School of Computer Science, China University of Geosciences(Wuhan), Wuhan 430078 , China
ZHANG Biao
School of Computer Science and Technology, Liaocheng University, Liaocheng 252000 , China
GAO Liang
State Key Laboratory of Intelligent Manufacturing Equipment and Technology, Huazhong University of Science and Technology,Wuhan 430074 , China
Abstract:
To address the issue of the lack of generalization capability of deep reinforcement learning in flexible job shop scheduling problems, a method combining curriculum learning and deep reinforcement learning was proposed. The training instance difficulty was dynamically adjusted, with an emphasis on enhancing the training of the most difficult instances, to adapt to different data distributions and avoid the forgetting problem during the learning process. Simulation test results demonstrate that the algorithm maintained decent performance on large-scale untrained problems and benchmark datasets. It achieves better performance on four large-scale untrained problems with two artificial distributions. Compared to exact methods and metaheuristic methods, for problem instances with larger computational complexity, it could rapidly obtain solutions of decent quality. Moreover, the algorithm can adapt to flexible job shop scheduling problems with different data distributions, exhibiting a relatively fast convergence speed and good generalization capability.
To address the issue of the lack of generalization capability of deep reinforcement learning in flexible job shop scheduling problems, a method combining curriculum learning and deep reinforcement learning was proposed. The training instance difficulty was dynamically adjusted, with an emphasis on enhancing the training of the most difficult instances, to adapt to different data distributions and avoid the forgetting problem during the learning process. Simulation test results demonstrate that the algorithm maintained decent performance on large-scale untrained problems and benchmark datasets. It achieves better performance on four large-scale untrained problems with two artificial distributions. Compared to exact methods and metaheuristic methods, for problem instances with larger computational complexity, it could rapidly obtain solutions of decent quality. Moreover, the algorithm can adapt to flexible job shop scheduling problems with different data distributions, exhibiting a relatively fast convergence speed and good generalization capability.
收稿日期:
2024-01-04
2024-01-04
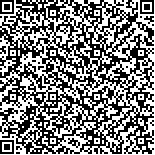