利用多层次特征融合网络的图像异常检测算法
2025,47(2):173-182
唐俊
安徽大学 电子信息工程学院, 安徽 合肥 230601
左金梅
安徽大学 电子信息工程学院, 安徽 合肥 230601
王科
安徽大学 互联网学院, 安徽 合肥 230039
张艳
安徽大学 电子信息工程学院, 安徽 合肥 230601
王年
安徽大学 电子信息工程学院, 安徽 合肥 230601
安徽大学 电子信息工程学院, 安徽 合肥 230601
左金梅
安徽大学 电子信息工程学院, 安徽 合肥 230601
王科
安徽大学 互联网学院, 安徽 合肥 230039
张艳
安徽大学 电子信息工程学院, 安徽 合肥 230601
王年
安徽大学 电子信息工程学院, 安徽 合肥 230601
摘要:
图像异常检测旨在识别并定位图像中的异常区域,针对现有算法中不同层次特征信息利用不充分的问题,提出了基于多层次特征融合网络的图像异常检测算法。通过使用融合了异常先验知识的伪异常数据生成算法,对训练集进行了异常数据扩充,将异常检测任务转化为监督学习任务;构建了多层次特征融合网络,将神经网络中不同层次特征进行融合,丰富了特征中的低层纹理信息和高层语义信息,使得用于异常检测的特征更具区分性;训练时,设计了分数约束损失和一致性约束损失,并结合特征约束损失对整个网络模型进行训练。实验结果表明,MVTec数据集上图像级检测接收机工作特性曲线下面积(area under the receiver operating characteristic, AUROC)平均值为98-7%,像素级定位AUROC平均值为97-9%,每区域重叠率平均值为94-2%,均高于现有的异常检测算法。
图像异常检测旨在识别并定位图像中的异常区域,针对现有算法中不同层次特征信息利用不充分的问题,提出了基于多层次特征融合网络的图像异常检测算法。通过使用融合了异常先验知识的伪异常数据生成算法,对训练集进行了异常数据扩充,将异常检测任务转化为监督学习任务;构建了多层次特征融合网络,将神经网络中不同层次特征进行融合,丰富了特征中的低层纹理信息和高层语义信息,使得用于异常检测的特征更具区分性;训练时,设计了分数约束损失和一致性约束损失,并结合特征约束损失对整个网络模型进行训练。实验结果表明,MVTec数据集上图像级检测接收机工作特性曲线下面积(area under the receiver operating characteristic, AUROC)平均值为98-7%,像素级定位AUROC平均值为97-9%,每区域重叠率平均值为94-2%,均高于现有的异常检测算法。
基金项目:
国家自然科学基金资助项目(61772032);安徽省重点研究与开发计划资助项目(202004a07020050,2022k07020006);安徽省高校自然科学研究重大资助项目(KJ2021ZD0004)
国家自然科学基金资助项目(61772032);安徽省重点研究与开发计划资助项目(202004a07020050,2022k07020006);安徽省高校自然科学研究重大资助项目(KJ2021ZD0004)
Image anomaly detection algorithm using multi-level feature fusion network
TANG Jun
School of Electronic Information Engineering, Anhui University, Hefei 230601 , China
ZUO Jinmei
School of Electronic Information Engineering, Anhui University, Hefei 230601 , China
WANG Ke
School of Internet, Anhui University, Hefei 230039 , China
ZHANG Yan
School of Electronic Information Engineering, Anhui University, Hefei 230601 , China
WANG Nian
School of Electronic Information Engineering, Anhui University, Hefei 230601 , China
School of Electronic Information Engineering, Anhui University, Hefei 230601 , China
ZUO Jinmei
School of Electronic Information Engineering, Anhui University, Hefei 230601 , China
WANG Ke
School of Internet, Anhui University, Hefei 230039 , China
ZHANG Yan
School of Electronic Information Engineering, Anhui University, Hefei 230601 , China
WANG Nian
School of Electronic Information Engineering, Anhui University, Hefei 230601 , China
Abstract:
Image anomaly detection aims to identify and locate the abnormal region in an image. To address the issue on the insufficient utilization of different-level feature information in the existing methods, an image anomaly detection method based on multi-level feature fusion network was put forward. By using the pseudo anomaly data generation algorithm incorporated with the anomaly prior knowledge, the anomaly data of the training set were augmented, and then the anomaly detection task was transformed into a supervised learning task. A multi-level feature fusion network was constructed to enriches the low-level texture information and high-level semantic information of features by fusing the different levels of features in the neural network, which could make the features used for anomaly detection more discriminative. In the training phase, the score constraint loss and the consistency constraint loss were designed and combined with the feature constraint loss to train the whole network model. Experimental results on the MVTec dataset showed that the proposed model could achieve 98.7% AUROC (area under the receiver operating characteristic) mean value in the detection task, 97.9% AUROC mean value in the pixel-wise localization task and 94.2% mean value of per-region-overlap in the localization task, which outperformed several existing anomaly detection approaches.
Image anomaly detection aims to identify and locate the abnormal region in an image. To address the issue on the insufficient utilization of different-level feature information in the existing methods, an image anomaly detection method based on multi-level feature fusion network was put forward. By using the pseudo anomaly data generation algorithm incorporated with the anomaly prior knowledge, the anomaly data of the training set were augmented, and then the anomaly detection task was transformed into a supervised learning task. A multi-level feature fusion network was constructed to enriches the low-level texture information and high-level semantic information of features by fusing the different levels of features in the neural network, which could make the features used for anomaly detection more discriminative. In the training phase, the score constraint loss and the consistency constraint loss were designed and combined with the feature constraint loss to train the whole network model. Experimental results on the MVTec dataset showed that the proposed model could achieve 98.7% AUROC (area under the receiver operating characteristic) mean value in the detection task, 97.9% AUROC mean value in the pixel-wise localization task and 94.2% mean value of per-region-overlap in the localization task, which outperformed several existing anomaly detection approaches.
收稿日期:
2022-12-08
2022-12-08
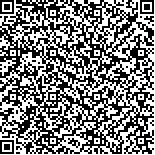